Webinar: Dynamic Deep Pixel Distribution Learning for Background Subtraction cnki
Speaker: Chenqiu Zhao received B.S. and M.S. degrees in software engineering from Chongqing University, Chongqing, China, in 2014 and 2017, respectively. He used to be a full-time Research Associate with the Institute for Media Innovation from 2017 to 2018, Nanyang Technological University, Singapore. He passed his Ph.D. defense in June 2022 at the Department of Computing Science, University of Alberta, and will join the Multimedia Research Centre, Department of Computing, the University of Alberta as a postdoc in August 2022. His current research interests include computer vision, video segmentation, pattern recognition, distribution learning, and deep learning. Abstract: Previous approaches to background subtraction usually approximate the distribution of pixels with artificial models. In this paper, we focus on automatically learning the distribution, using a novel background subtraction model named Dynamic Deep Pixel Distribution Learning (D-DPDL). In our D-DPDL model, a distribution descriptor named Random Permutation of Temporal Pixels (RPoTP) is dynamically generated as the input to a convolutional neural network for learning the statistical distribution, and a Bayesian refinement model is tailored to handle the random noise introduced by the random permutation. Because the temporal pixels are randomly permutated to guarantee that only statistical information is retained in RPoTP features, the network is forced to learn the pixel distribution. Moreover, since the noise is random, the Bayesian theorem is naturally selected to propose an empirical model as a compensation based on the similarity between pixels. Evaluations using standard benchmark demonstrates the superiority of the proposed approach compared with the state-of-the-art, including traditional methods as well as deep learning methods. Click here to join or watch the recording. |
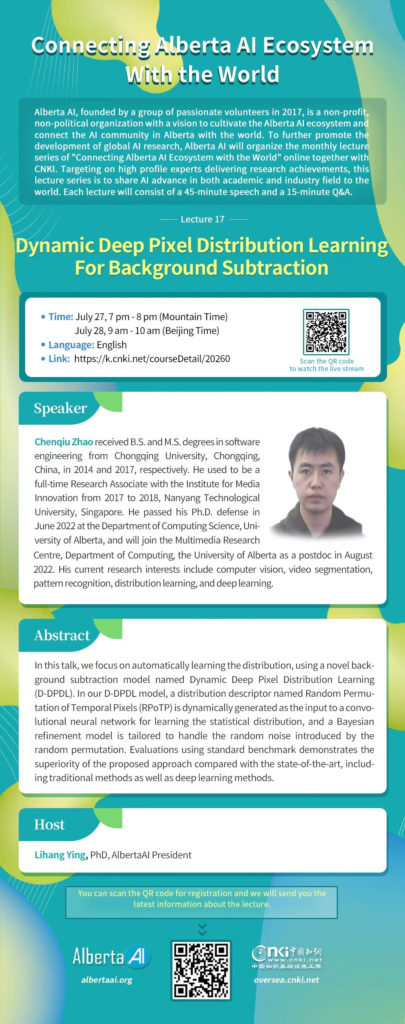